Optimizing Supplier Selection through Advanced Data Science and Risk Modeling: A Comprehensive Guide for Supply Chain Professionals
Advanced data science and risk modeling can significantly improve supplier selection processes, enabling supply chain professionals to make more informed decisions. By incorporating these innovative techniques, organizations can optimize their supplier portfolios, mitigate potential risks, and build more resilient supply chains in a complex global environment.
Introduction
The process of selecting and managing suppliers is a critical aspect of supply chain management, as it directly impacts an organization's efficiency, profitability, and resilience. As global supply chains become more complex and interconnected, supply chain professionals must adopt innovative approaches to optimize supplier selection. This article will discuss how advanced data science and risk modeling can enhance supplier selection processes, providing organizations with the tools to make more informed decisions and build more resilient supply chains.

Leveraging advanced data science supplier selection
Data science, which involves the use of algorithms, statistical models, and machine learning techniques to extract insights from data, can significantly improve supplier selection processes. Some key applications of data science in supplier selection include:
- Data Integration: By consolidating data from various sources, such as financial reports, quality metrics, and industry benchmarks, organizations can create comprehensive supplier profiles that facilitate more accurate and informed decision-making.
- Predictive Analytics: Data science techniques can analyze historical data to identify patterns and trends that may indicate potential risks or opportunities related to supplier performance, enabling organizations to make proactive decisions.
- Supplier Segmentation: Machine learning algorithms can segment suppliers based on factors such as risk profiles, performance metrics, and strategic importance, allowing organizations to prioritize and allocate resources more effectively.
- Optimization Models: Data-driven optimization models can help organizations identify the optimal mix of suppliers to meet their specific objectives, such as minimizing costs, maximizing quality, or achieving a certain level of supply chain resilience.
Incorporating Risk Modeling in Supplier Selection
Effective supplier selection requires a thorough understanding of the potential risks associated with each supplier. By integrating risk modeling into the supplier selection process, organizations can better assess and mitigate these risks. Some essential components of risk modeling in supplier selection include:
- Risk Assessment Framework: Develop a comprehensive risk assessment framework that considers various factors, such as financial stability, cybersecurity posture, and geopolitical risks, to evaluate potential suppliers.
- Quantitative Risk Scoring: Assign numerical risk scores to suppliers based on their performance across the different risk factors identified in the risk assessment framework. This enables organizations to easily compare and prioritize suppliers based on their risk profiles.
- Scenario Analysis: Conduct scenario analyses to assess the potential impact of various risk events on the organization's supply chain, helping to identify vulnerabilities and inform risk mitigation strategies.
- Risk Mitigation Planning: Develop and implement risk mitigation plans for each supplier based on their risk scores and the results of scenario analyses. Regularly review and update these plans to ensure their effectiveness and relevance.
Conclusion
The application of advanced data science and risk modeling techniques can significantly enhance the supplier selection process, empowering supply chain professionals to make more informed and strategic decisions. By leveraging these innovative approaches, organizations can optimize their supplier portfolios, mitigate potential risks, and build more resilient supply chains in an increasingly complex and interconnected global environment.
Latest Articles
A secure, more resilient supply chain is here.

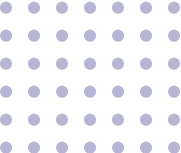